How to Ensure Data Integrity: 4 Processes for Your Data Lake or Data Warehouse
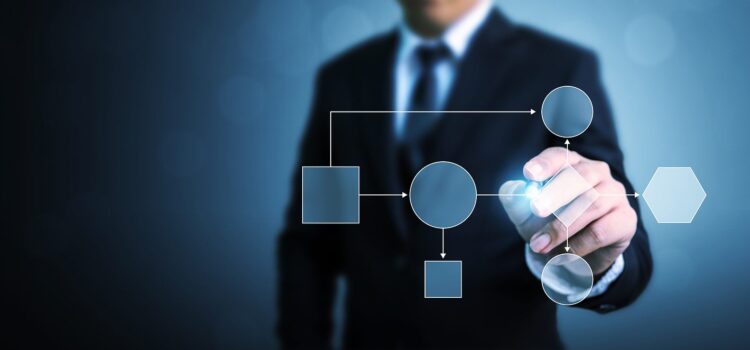
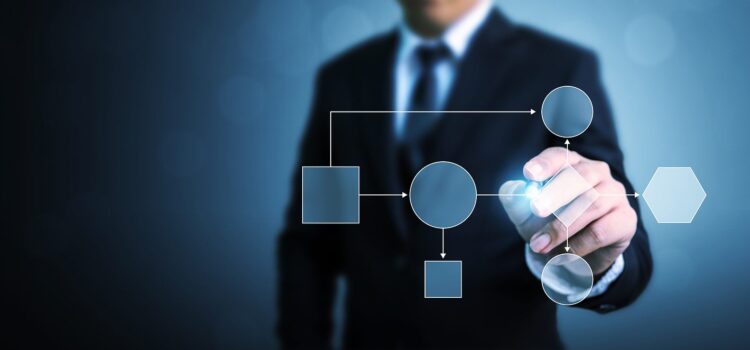
How can you ensure that the data in your data lake or data warehouse is trusted? Addressing that question is an important part of making sure that you have quality data. Ensuring your data is clean, validated, organized, and available in near-real or real-time is crucial to run an effective solution.
Here are four processes that will help make your data reliable.
1. Catalog the Data
All data must be cataloged so that it can be identified, searched, and found. This requires an investment into a cataloging capability to ensure that all data in your data lake or data warehouse is known, cataloged, tagged, curated, data lineage details revealed, and taxonomies defined. Paramount to the cataloging process is the creation of a data dictionary. The discovery of the data should be an automated process and should always be up to date.
As part of the cataloging of data, there is typically a tagging process where data stewards can label and categorize information about data which supports the search and discovery process for users. And further, tagging can also be used to secure specific types of data such as PII data. Integration between the data tagging component and security policies is advantageous to associate and execute security policies for specific types of data automatically. The process of tagging can be done either manually, by data stewards, or through auto-tagging. Auto-tagging functionality is performed through a machine learning capability that improves over time as it learns from the previous tags and updates by data stewards. Most of the better data catalog solutions have both manual and ML-based tagging. Most data lakes and data warehouses have configurable security policies that establish specific rules for all data types and how to handle each type.
2. Data Governance
Data governance refers to the people, processes, policies, procedures, technology/tools, standards, roles and responsibilities of governing parties for all data assets—and is essential for an effective solution. Components of data governance include data quality, data stewardship, master data management, data timeliness, and data accuracy.
3. Auditing and Access Management
Once you have a data catalog and security policies defined for various data types and tags, and data lineage is established, then the “trust in the data” goal is almost complete. The next step is to audit and log access to the data. Most Data Lakes and Warehouses have audit capabilities that can simply be enabled that log access to any data. As a matter of principle, all data access is logged, and if a user tries to access data that they do not have access privileges for then this will be flagged in the audit file which is in accordance with expectations from Security teams, Audit authorities, and compliance mandates.
4. Reporting Mechanism and Processes
Once data has been tagged and certified either through data stewards or artificial intelligence, it is ready for reporting. Reports can be certified in several ways, usually by data stewards or business analysts or as documented in the Governance policies. Ultimately, reports can be watermarked with a “certified” tag, and grouping certified reports together by function is also possible.
Data and reports alike need to be secured—encrypted at rest, in motion, and secured through policies and rules. Security of data and reports can benefit from using Role-based Access Control (RBAC). RBAC restricts access based upon a person’s role within an organization. RBAC has many potential advantages including operational efficiency, enhanced compliance, reduced costs, better security by decreasing breaches and data leakages.
Read more about how to overcome challenges facing Enterprise Architects and download our new whitepaper.